Machine learning: The knockout needed to beat ad fraud
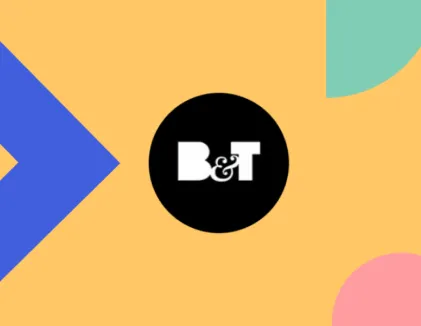
âOriginally appearing in B&T MAGAZINE, TrafficGuardâs COO, Luke Taylor, explores how machine learning could be just whatâs needed to beat ad fraud.
With a recent report from the Association of National Advertisers (ANA) suggesting that spend lost through ad fraud is decreasing, you may be forgiven for thinking that weâve overcome the worst, and are on the path to adland victory. After all, the industry has indeed made some strong headway over the past year, with greater cooperation and a renewed commitment to the right technologies and processes proving that effective tools do deter would-be fraudsters from investing in the cause.
That said, we cannot underestimate the sheer scale of ad fraud today â according to our research, fraudsters swindled a total of US$18 billion last year from advertisers in APAC alone. Nor should we underestimate the fraudsters themselves.
The reality is that we are in an arms race against fraud operations that are run like businesses â they invest in R&D and innovate to ensure that they continue to make a profit and when they are faced with threats, like any business, they adapt. And as we move our budgets to new platforms â including mobile apps and on-demand video â these operations are already innovating ways to exploit loopholes and siphon off your ad spend.
AdTech has been late to the game and the temptation is to play catch-up by closing off old holes in the ecosystem. But while patching these old holes, fraudsters are already looking ahead for new ways to defraud the advertisers. This is the reality of a reaction based approach.
Instead of reacting as new variations of fraud tactics and exploits evolve, it is time for a proactive approach â time for advertisers to get ahead of ad fraud. This may seem fantastical in a time when ad fraud makes headlines on a daily basis, but it is possible when we draw inspiration from specialist security solutions that leverage big data and machine learning.
First though, what do we mean by machine learning?
In a nutshell, machine learning is a subset of artificial intelligence that extracts patterns and identifies relationships from data, then expresses these as formulas that can be applied to new datasets. Put simply, this essentially means that over time, as the data changes, the technology learns to recognise new trends and autonomously action improvements without the need to explicitly program it.
The term âmachine learningâ has been making the rounds of late, supporting all sorts of tech â from virtual personal assistants to online customer support, healthcare research and facial recognition. Digital advertising, which generates swathes of data every second, is no exception. In fact, according to the same TrafficGuard study, machine learning is expected to save app developers and advertisers across Asia Pacific US$3.5 billion by 2022, compared to US$576 million in 2018.
Of course, thatâs not to say that machine learning is something to set and forget. The technology is indeed complex, and requires constant training, testing and validation in order to be effective. Data processed by machine learning models need rigorous preparation. Infrastructure and storage need to be scalable and task appropriate. So there are certainly a number of barriers to entry.
Want to understand more about machine learning in fraud prevention? Download our eBook.
But where does it fit into the ad fraud mitigation mix? To better understand machine learningâs capabilities, letâs consider the insufficiencies of current fraud prevention tools.
Blacklists, for one â whereby advertisers block traffic coming from IP addresses that have previously supplied invalid traffic â can be very easily circumvented by fraudsters by simply swapping their IP addresses. Not to mention that blacklisting can unwittingly block genuine engagement with the same sweeping brush.
Rule-based mitigation (i.e. block traffic that acts in this way), while more effective, is ultimately reactive. Rules only work once the case against a certain fraudulent tactic has been built â meaning theyâre completely useless against what our most recent research has dubbed Zero Day Ad Fraud (new and emerging types of fraud) until budgets have been severely impacted. As fraud evolves, rule libraries get increasingly complex, cumbersome to manage and error prone.
However, machine learning, with its ability to review multiple variables and dimensions of any given dataset, is able to look beyond two-dimensional indicators â typically the limit of what a human can perceive. Instead, capable of processing large and high dimensional data, machine learning models can detect anomalies and cluster transactions to facilitate the discovery of new fraud tactics and earlier indicators of existing tactics.
Crucially, this means that instead of waiting until a fraudulent tactic has reached mass scale to be able to reactively diagnose it as fraud, it can be invalidated reliably, before it drains ad budgets.
Fraud isnât the only part of the ecosystem that is evolving. As ad tech evolves and consumer behaviour changes, even genuine traffic patterns change â which is another complexity of managing rules. For fraud prevention to be effective, it needs to adapt to these changes and audience nuances to ensure legitimate traffic isnât removed in the fraud mitigation process. Instead of reacting to fraud as new tactics emerge with new rules, machine learning can be part of a proactive defence that is tactic-agnostic, more accurate and able to stop fraud before the fraudster gets paid.
The battle will only intensify from here on out. As digital ad spend continues to rise globally, adland will become increasingly arable for fraud. And for all the industry optimism of late, the truth is the industry still has a long way to go before itâs fraud-free. Recent takedowns have required an unprecedented level of collaboration and substantial funds to stop just a handful of relatively unsophisticated fraud operations.
To combat sophisticated fraud â the type of fraud that flies under the radar of traditional fraud prevention â a more proactive approach is required. By using machine learning, we take a sustainable approach to fraud prevention, protecting ad spend from tomorrowâs fraud, as well as todayâs.
To learn more about why machine learning is crucial in fraud prevention, download our eBook.
â
Get started - it's free
You can set up a TrafficGuard account in minutes, so weâll be protecting your campaigns before you can say âsky-high ROIâ.
Subscribe
Subscribe now to get all the latest news and insights on digital advertising, machine learning and ad fraud.