Maximising ROAS in eCommerce with Multi-Channel Bot Detection
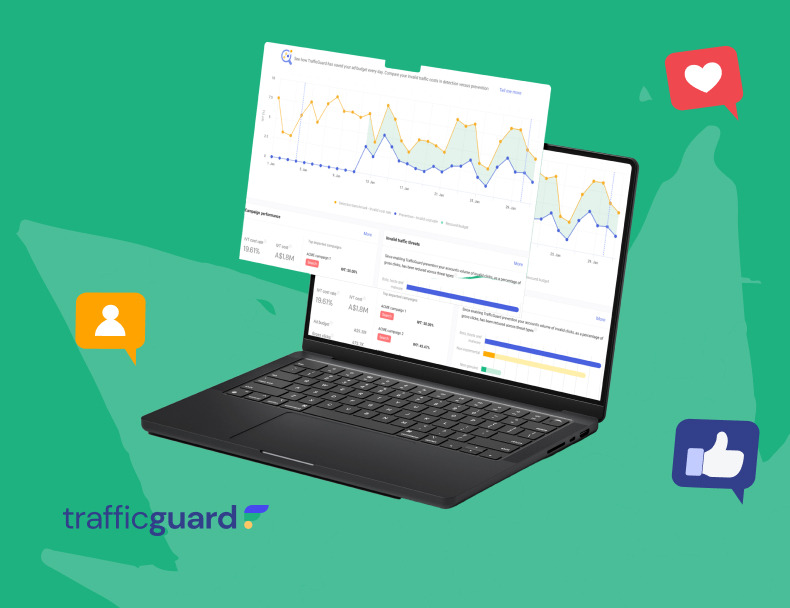
As businesses compete for consumer attention across platforms like Google Ads, Facebook, and TikTok, fraudsters exploit these same systems to siphon ad budgets through fraudulent activities. Bot detection has become increasingly critical as bots target campaigns with tactics that inflate metrics but deliver no real value.
The types of bots impacting eCommerce are more diverse than other sectors, as real users generally have an immediate purchase intent.
Click farms, for instance, generate fake clicks on pay-per-click (PPC) ads, rapidly draining budgets while mimicking legitimate user behaviour. Scraper bots extract pricing and product information, potentially driving up competition in eCommerce ads, while corrupting analytics. Even more damaging are inventory hoarding bots, which fill shopping carts without completing purchases, creating artificial scarcity and frustrating genuine customers. These bots target key eCommerce channels such as shopping, search, social, display, and even event-driven platforms like PMax, each presenting unique vulnerabilities.
Performance metrics for your ad campaigns are impacted as a result. For example, click-through rates (CTR) become inflated, masking the true engagement levels. Conversion rates inevitably drop as fraudulent traffic fails to complete meaningful actions, and customer acquisition costs (CAC) skyrocket as budgets are wasted on invalid clicks.
The knock-on effect of this undermines return on ad spend (ROAS) metrics and skews strategic marketing decisions that are made as a result of inaccurate campaign performance figures.
A Multi-Point Fraud Detection System
ad fraud is a multifaceted problem that requires a comprehensive solution. TrafficGuard’s bot detection architecture is designed to operate at every stage of the advertising lifecycle impression, click, and post-click. This way fraudulent activity is caught and mitigated before it can impact performance.
At the core of TrafficGuard’s Multi-Point Fraud Detection System is real-time monitoring. The platform evaluates traffic as it interacts with your campaigns, using a combination of device-level data, behavioural analytics, and advanced machine learning to identify anomalies. This begins with impression tracking, where bots often mimic genuine ad views. By cross-referencing impressions against historical patterns and known fraud databases, TrafficGuard can instantly detect invalid traffic sources.
Click fraud protection is equally important as bots frequently exhibit tell-tale behaviours, such as high click velocity (rapid, repeated clicks) or unusual geographic dispersion (traffic originating from multiple unrelated regions). TrafficGuard’s system evaluates these patterns, flagging suspicious activity and blocking it before it can inflate costs. Post-click analysis adds another layer of protection, monitoring user behaviour on landing pages. For instance, bots may navigate erratically or leave a page within seconds, behaviours that are automatically identified as fraudulent.
TrafficGuard also leverages sophisticated technologies, including IP reputation databases and device fingerprinting. With IP reputation databases, the platform will block traffic from known malicious sources, while device fingerprinting creates unique identifiers for devices, which prevents fraudsters from using rotating IPs to evade detection. These techniques, combined with seamless API integrations, ensure that TrafficGuard can provide an end-to-end ad fraud detection platform.
Advanced Implementation
The technical implementation of the platform goes beyond surface-level detection to address the complexities of modern ad fraud prevention. For client-side monitoring, JavaScript tags are embedded on the website, allowing the platform to collect granular data on user interactions. Meanwhile, tracking templates are added to eCommerce ads to monitor key metrics such as browser type, interaction timestamps, and session duration. Together, these components provide a detailed profile of user behaviour and enhance fraud detection capabilities.
Server-side log analysis complements this approach by examining traffic patterns at scale. Logs provide valuable insights into anomalies like spikes in click volume from single IPs or discrepancies in geographic targeting. It is by combining these methods, that TrafficGuard builds a comprehensive view of traffic quality, enabling precise click fraud detection.
Machine learning is the nucleus of TrafficGuard’s effectiveness; the platform employs both supervised and unsupervised models to detect fraud. Supervised models are trained on historical data to recognise known fraud patterns, while unsupervised models identify emerging threats by clustering unfamiliar behaviours. This approach from both ends make sure that TrafficGuard remains effective against evolving click fraud tactics.
Key indicators such as click velocity, session depth (the number of pages visited), and bounce rates are pillar metrics that get analysed to differentiate legitimate users from bots. Also, predictive analytics enable the platform to anticipate fraud before it occurs, using historical data to block suspicious traffic sources before they start hampering your data.
Unified Cross-Channel Analysis for Holistic Protection
ad fraud is not limited to a single platform. It often spans multiple platforms, making it essential to analyse traffic in a unified manner. TrafficGuard’s cross-channel capabilities are powered by a real-time data streaming architecture, enabling the platform to process vast amounts of traffic data from search, social, and programmatic channels in parallel.
This all funnel approach allows for advanced techniques such as graph-based analysis, which identifies coordinated bot networks. For example, bots operating across different platforms may share IPs or behavioural traits, patterns that can only be detected through comprehensive cross-channel analysis. By correlating data in real time, TrafficGuard detects these patterns, providing a level of fraud detection that standalone tools cannot match.
Mitigating Fraud and Optimising ROAS
However, fraud detection is only half the battle. The other half is mitigation and it is here the real value lies. TrafficGuard employs a range of strategies to neutralise fraudulent traffic in real-time. Dynamic IP blocking, for instance, prevents bots from re-engaging with your campaigns after being flagged. Rate-limiting strategies ensure that high-frequency interactions are curtailed, further reducing the impact of click fraud and other automated fraud mechanisms.
TrafficGuard also uses browser fingerprinting and behavioural biometrics which take it up a notch on protection. They act as an early warning system, allowing TrafficGuard to detect and block bots before they interact with real ads.
Finally, budgets that would have been wasted on bots are redirected to genuine users, resulting in higher conversion rates and lower customer acquisition costs. This clean data also enables more accurate attribution modelling, helping marketers optimise their campaigns with confidence.
Learn how TrafficGuard can secure your ROAS, request a demo or start a free trial today.
Get started - it's free
You can set up a TrafficGuard account in minutes, so we’ll be protecting your campaigns before you can say ‘sky-high ROI’.
Subscribe
Subscribe now to get all the latest news and insights on digital advertising, machine learning and ad fraud.